The issue of AI is of ever-increasing relevance (particularly to data privacy and compliance professionals), as the number of AI solutions grows exponentially and AI use-cases become ever more astonishing.
At the same time, regulators and law makers around the world are scrambling to govern a broad, exceptionally fast-paced and complex area of technology. This has left organisations (and their data privacy, legal and/or compliance functions) struggling to stay on top of rapid developments and ever-changing regulations.
This page sets out an overview of key considerations for data privacy professionals tasked with tackling AI regulation and governance projects.
“If used well, AI has the potential to make organisations more efficient, effective and innovative. However, AI also raises significant risks for the rights and freedoms of individuals, as well as compliance challenges for organisations.”
ICO (UK) - guidance on data protection and AI (2023)
Key regulatory compliance topics: compliant implementation of AI
Data Privacy and AI |
1) Identify the specific legal analysis required for each part of the process: e.g. can the development phase of the AI system can be separated from the operational/deployment phase? Do they need to be assessed separately? 2) Define a purpose for any processing of personal data, which must be done from the very outset of a project, and recorded in the register of processing activities. 3) Determine your roles: data controller (perhaps a supplier who initiates the development of a system); data processor (perhaps a service provider used solely to collect and process data to train an AI model), or even joint controllers (e.g. where several controllers provide data to train an AI model for a jointly defined objective). 4) Assess lawful processing conditions, ensuring that the processing is lawful, focusing particularly on data collection and any additional tests necessary for the proper re-use of personal data for a new purpose (remembering to distinguish between: (i) personal data used to train an AI model; and (ii) personal data used as an input to an AI model). 5) Complete a data protection impact assessment (DPIA) where higher risk processing is expected, considering specific risks such as automated discrimination caused by algorithmic bias, erroneous fictional content created about a real person, and individuals losing control of their data (particularly through data harvesting/web-scraping). 6) Implement rigorous management and monitoring of data usage to ensure privacy by design, focusing on data collection, monitoring and updating data, data retention, data security, and documenting decisions made in respect of data usage.
|
Transparency |
In addition to general transparency (privacy notice) obligations under data privacy laws, it is also important to consider AI-specific transparency. In the context of AI, transparency amounts to building on the obligations that likely already exist under a particular jurisdiction’s data privacy laws, by making AI decision-making clear, and sufficiently explaining decisions and outcomes in a meaningful, comprehensible way. In addition, it is worth considering specific transparency obligations relating to automated decision-making, such as the obligations under the GDPR to provide information on the existence of automated decision-making, including profiling, alongside meaningful information about the logic involved, as well as the significance and the envisaged consequences of such processing for the data subject.
|
Privacy by design |
Focus on: 1) implementing proportional technical and organisational measures to ensure that individuals’ data privacy rights are upheld at each stage of the processing of their personal data; 2) assessing and documenting risks and impacts that an AI project or system could have on relevant individuals, both at the outset and throughout the rest of the project’s lifecycle; and 3) thinking of so-called “ethics by design”, identifying particular requirements that can ensure the fair and ethical use of individuals’ data within AI systems.
|
Accountability |
Focus on: 1) assessing the risks posed to individuals by the AI system; 2) understanding and set out how those risks can and will be effectively mitigated (making use of existing processes such as DPIAs); and 3) documenting this process and the effect that this risk mitigation has on the AI system, creating a demonstrable history of compliance.
|
Fairness |
While of greater relevance to engineers, fairness is also an important concept for decisionmakers and data privacy professionals. Fairness is naturally already a well-understood concept in the world of data privacy but, in the context of AI, fairness may also bring into focus issues such as accuracy, discrimination, data ethics, algorithmic fairness, and other (e.g. sector-specific) concepts of fairness. Certain organisational approaches (such as completing DPIAs, adhering to data protection by design and default, and implementing governance measures) will also improve fairness in an AI project/system.
|
Profiling and automated decision-making |
While data privacy legislation is generally technology neutral, and does not include provisions specifically addressing AI, the general concepts of profiling and automated decision-making are arguably the most relevant when it comes to the crossover between data privacy law and AI. Many pieces of privacy legislation around the world provide individuals with the right not to be subject to automated decision-making or profiling which has significant effects. It is likely that automated decision-making, and possible profiling, will be key parts of any data processing within a meaningful AI system, which makes compliance with any underlying data privacy laws particularly pertinent. Generally, organisations should exercise caution and, in following the GDPR’s position, ensure that: 1) any profiling or automated decision-making involving personal data is undertaken in ways which do not ignore the rights and interests of affected individuals; and 2) particularly in respect of profiling or automated decisions involving more sensitive categories of personal data, discriminatory effects are sufficiently prevented.
|
DPIAs |
The threshold around the world for having to complete a data protection impact assessment (or the equivalent) (DPIA) is – broadly speaking - where data processing poses a higher risk to individuals’ rights and freedoms, or where there is significant automated processing or profiling of individuals. AI is by nature difficult to deploy in a transparent and safe manner, and additional safeguards (such as DPIAs and related mitigation measures) will very often be necessary (and probably always best-practice).
|
Data minimisation / data retention |
An AI system will generally be built, trained and/or operated on very large sets of underlying data. The risks of breaching legal and regulatory obligations relating to data minimisation and data retention are significant, and steps should be taken to collect only what personal data is genuinely necessary, and to identify suitable (and lawful) retention and deletion periods.
|
“AI has become hungry for data, and this hunger has spurred data collection, in a self-reinforcing spiral: the development of AI systems based on machine learning presupposes and fosters the creation of vast data sets… The integration of AI and big data can deliver many benefits for the economic, scientific and social progress. However, it also contributes to risks for individuals…”
European Parliament – Study on the impact of the GDPR on AI
Finding and mapping personal data in AI systems: where has it all gone?
Within any AI project lifecycle, there is a wide range of ways in which the processing of personal data can become an issue. While personal data may be found in any location and at any stage of any AI-related project, the following table sets out the most common sources of personal data in AI projects and/or systems.
Where to find personal data within AI systems |
|
Underlying datasets |
The principal source of personal data is likely to be the very information on which an AI system is trained (i.e. the underlying dataset(s) fed into the system). Given the very large scale of these types of datasets, the possible data privacy implications are numerous.
|
Direct inputs (from human users) |
If an AI system requires or allows direct data inputs from users (which is particularly relevant in the case of Generative AI (e.g. by way of a query asked of an AI chatbot)), then any such input may well include personal data, which will then be processed by the system.
|
Publicly available data (from the internet) |
Generative AI, in the search for a response to a query, may obtain personally identifiable information from a publicly available source (e.g. the internet). Under most key data privacy regimes, such data is likely to still be treated as identifiable personal data.
|
Fine-tuning (transfer learning) |
This is the process by which an existing pre-trained computer model learns from (i.e. is trained on) new data that is added in order to finetune its functionality. Any new data may include personal data and/or data from which personal data may be inferred.
|
Artificial hallucination |
Where a Generative AI system provides unexpected, non-sensical responses, this process is often described as artificial hallucination, and any data generated as a result of such a process could include completely unforeseen instances or categories of personal data.
|
AI inferred data |
If an AI system can make inferences about an identifiable individual, based on various sources or correlations between different datasets, those inferences will be personal data and subject to data privacy laws. The concept of (and risks associated with) profiling may also be relevant.
|
AI generated outputs |
The data which is generated by an AI system (e.g. in response to a direct query), could naturally include personal data which would be subject to laws in the same way as any other form of personal data.
|
Key global AI governance frameworks, compared
This table sets out a comparative overview of AI governance frameworks in key jurisdictions around the world. This information is taken from detailed underlying legal analysis available within Rulefinder Data Privacy’s AI Regulation & Governance module. Note: we will be continuously monitoring and adding new jurisdictions over the coming weeks and months.
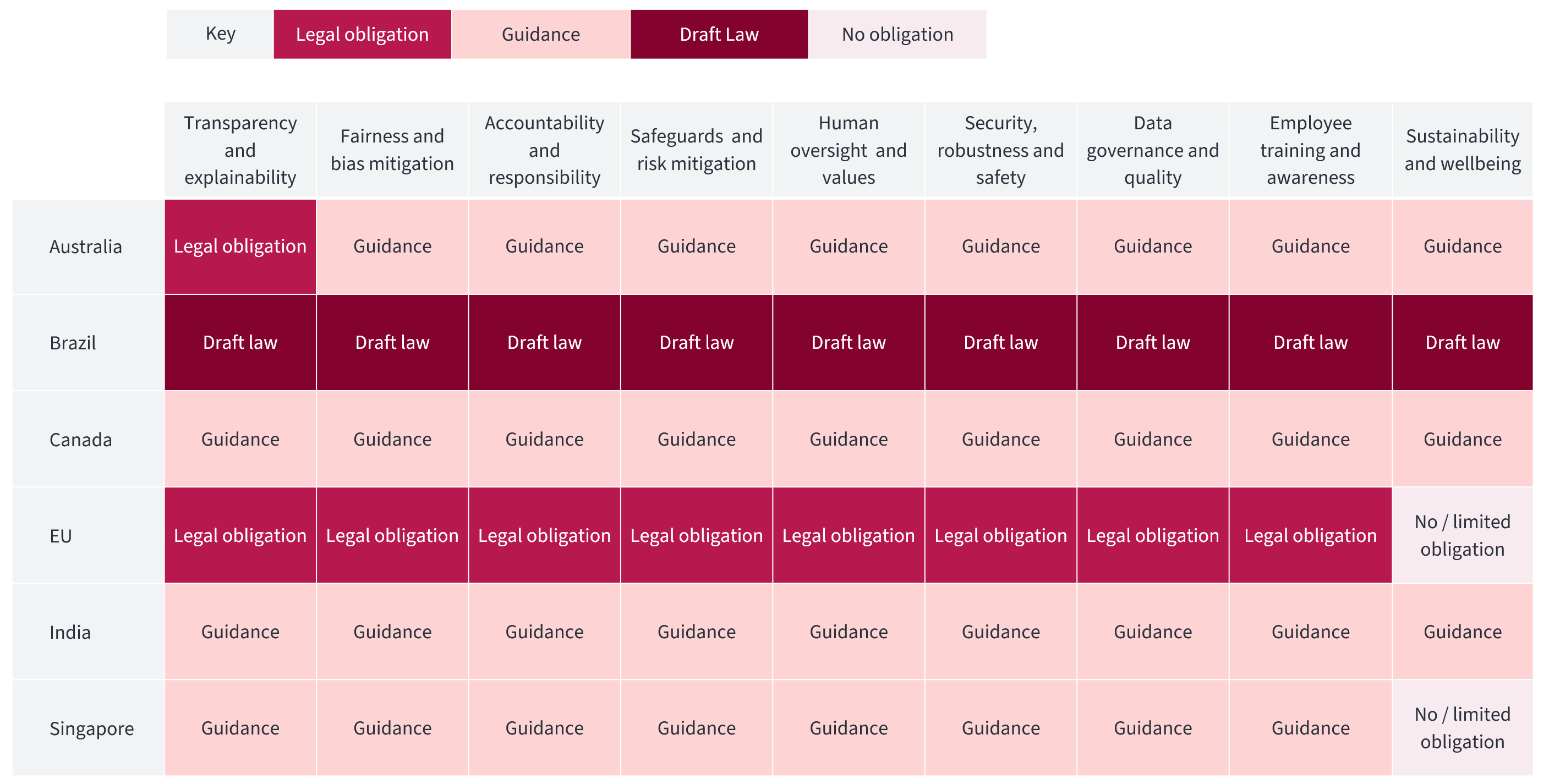
Want to find out more?
Rulefinder Data Privacy subscribers hear about these and other privacy law developments as soon as we cover them
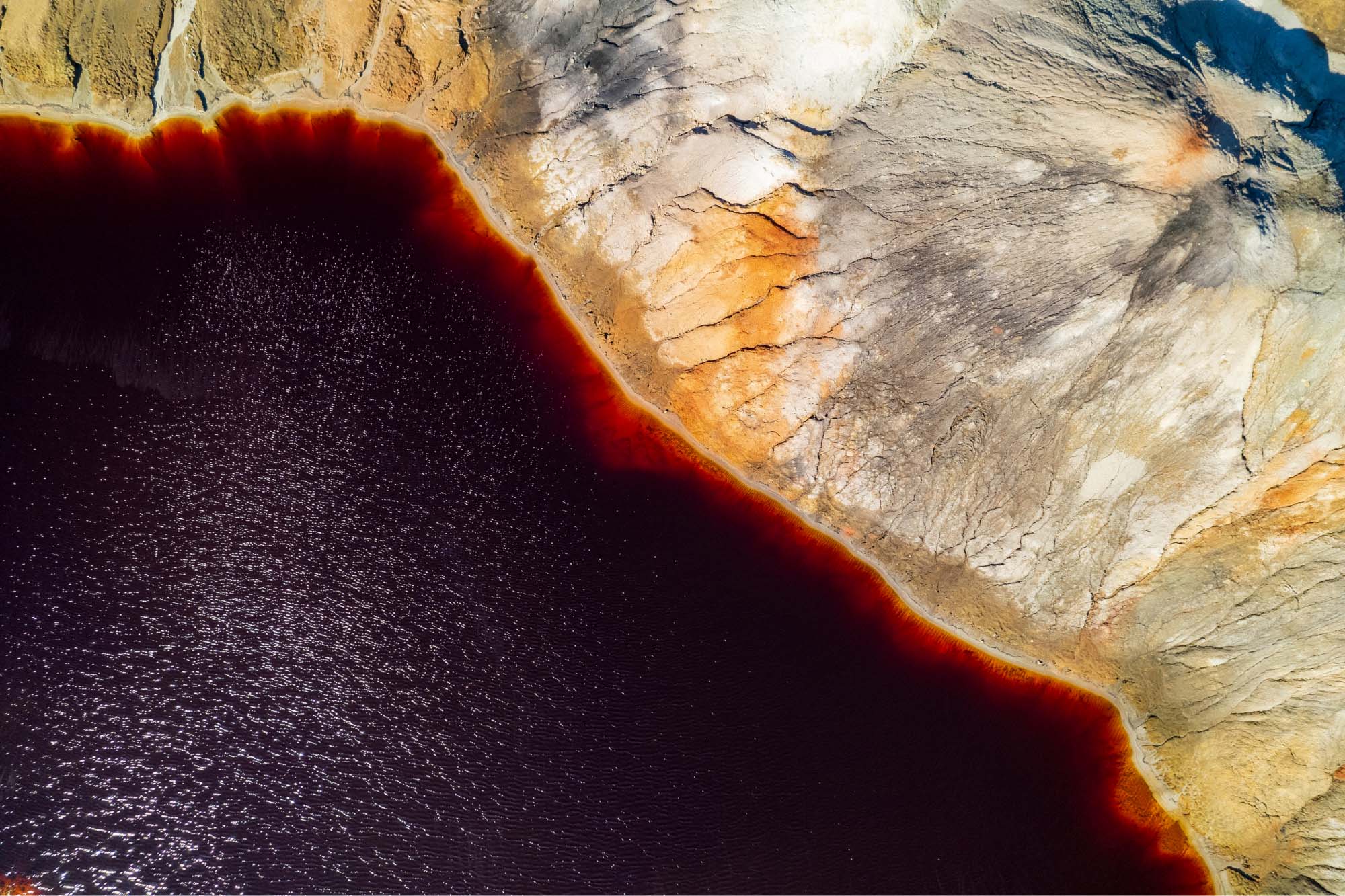